braindecode.models.ShallowFBCSPNet#
- class braindecode.models.ShallowFBCSPNet(n_chans=None, n_outputs=None, n_times=None, n_filters_time=40, filter_time_length=25, n_filters_spat=40, pool_time_length=75, pool_time_stride=15, final_conv_length='auto', conv_nonlin=<function square>, pool_mode='mean', activation_pool_nonlin: ~torch.nn.modules.module.Module = <class 'braindecode.models.modules.SafeLog'>, split_first_layer=True, batch_norm=True, batch_norm_alpha=0.1, drop_prob=0.5, chs_info=None, input_window_seconds=None, sfreq=None)[source]#
Shallow ConvNet model from Schirrmeister et al (2017) [Schirrmeister2017].
Model described in [Schirrmeister2017].
- Parameters:
n_chans (int) – Number of EEG channels.
n_outputs (int) – Number of outputs of the model. This is the number of classes in the case of classification.
n_times (int) – Number of time samples of the input window.
n_filters_time (int) – Number of temporal filters.
filter_time_length (int) – Length of the temporal filter.
n_filters_spat (int) – Number of spatial filters.
pool_time_length (int) – Length of temporal pooling filter.
pool_time_stride (int) – Length of stride between temporal pooling filters.
final_conv_length (int | str) – Length of the final convolution layer. If set to “auto”, length of the input signal must be specified.
conv_nonlin (callable) – Non-linear function to be used after convolution layers.
pool_mode (str) – Method to use on pooling layers. “max” or “mean”.
activation_pool_nonlin (callable) – Non-linear function to be used after pooling layers.
split_first_layer (bool) – Split first layer into temporal and spatial layers (True) or just use temporal (False). There would be no non-linearity between the split layers.
batch_norm (bool) – Whether to use batch normalisation.
batch_norm_alpha (float) – Momentum for BatchNorm2d.
drop_prob (float) – Dropout probability.
chs_info (list of dict) – Information about each individual EEG channel. This should be filled with
info["chs"]
. Refer tomne.Info
for more details.input_window_seconds (float) – Length of the input window in seconds.
sfreq (float) – Sampling frequency of the EEG recordings.
- Raises:
ValueError – If some input signal-related parameters are not specified: and can not be inferred.
FutureWarning – If add_log_softmax is True, since LogSoftmax final layer: will be removed in the future.
Notes
If some input signal-related parameters are not specified, there will be an attempt to infer them from the other parameters.
References
[Schirrmeister2017] (1,2)Schirrmeister, R. T., Springenberg, J. T., Fiederer, L. D. J., Glasstetter, M., Eggensperger, K., Tangermann, M., Hutter, F. & Ball, T. (2017). Deep learning with convolutional neural networks for EEG decoding and visualization. Human Brain Mapping , Aug. 2017. Online: http://dx.doi.org/10.1002/hbm.23730
Examples using braindecode.models.ShallowFBCSPNet
#
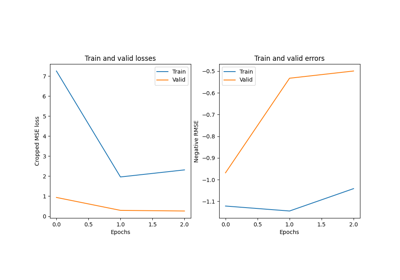
Convolutional neural network regression model on fake data.
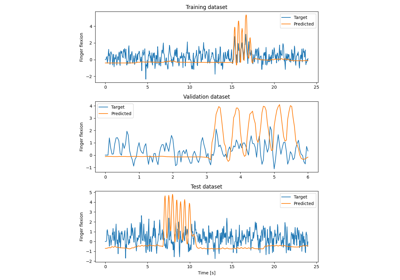
Fingers flexion cropped decoding on BCIC IV 4 ECoG Dataset
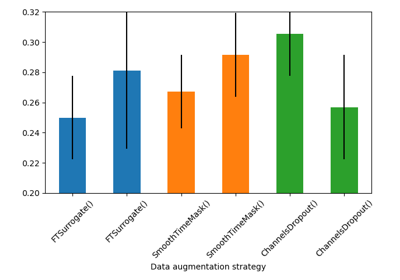
Searching the best data augmentation on BCIC IV 2a Dataset
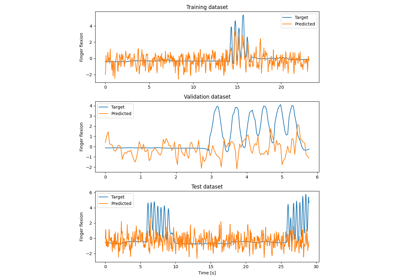
Fingers flexion decoding on BCIC IV 4 ECoG Dataset