braindecode.preprocessing.preprocess#
- braindecode.preprocessing.preprocess(concat_ds, preprocessors, save_dir=None, overwrite=False, n_jobs=None)[source]#
Apply preprocessors to a concat dataset.
- Parameters:
concat_ds (BaseConcatDataset) – A concat of BaseDataset or WindowsDataset datasets to be preprocessed.
preprocessors (list(Preprocessor)) – List of Preprocessor objects to apply to the dataset.
save_dir (str | None) – If a string, the preprocessed data will be saved under the specified directory and the datasets in
concat_ds
will be reloaded with preload=False.overwrite (bool) – When save_dir is provided, controls whether to delete the old subdirectories that will be written to under save_dir. If False and the corresponding subdirectories already exist, a
FileExistsError
will be raised.n_jobs (int | None) – Number of jobs for parallel execution. See joblib.Parallel for a more detailed explanation.
- Returns:
Preprocessed dataset.
- Return type:
Examples using braindecode.preprocessing.preprocess
#
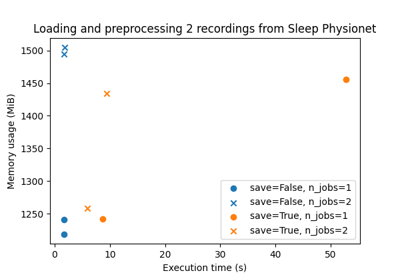
Benchmarking preprocessing with parallelization and serialization
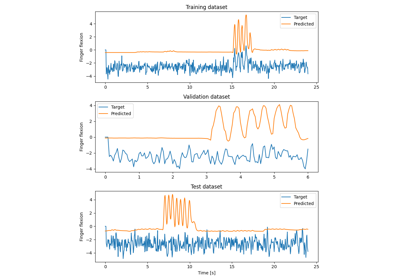
Fingers flexion cropped decoding on BCIC IV 4 ECoG Dataset
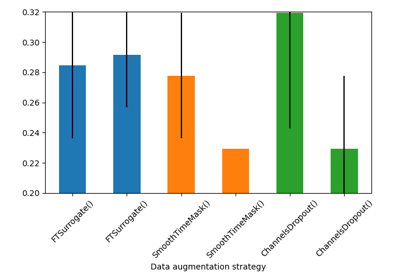
Searching the best data augmentation on BCIC IV 2a Dataset
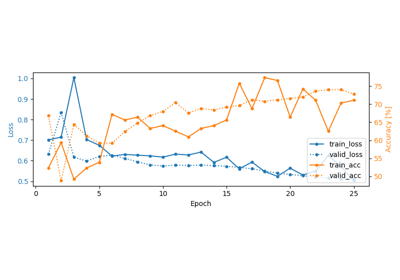
Self-supervised learning on EEG with relative positioning
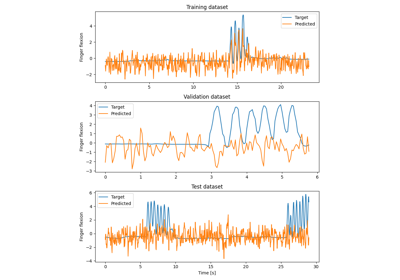
Fingers flexion decoding on BCIC IV 4 ECoG Dataset
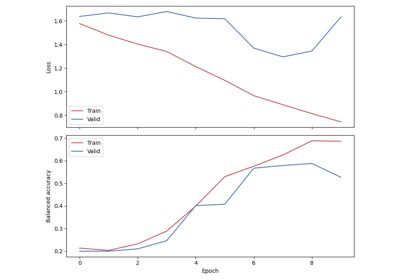
Sleep staging on the Sleep Physionet dataset using Chambon2018 network
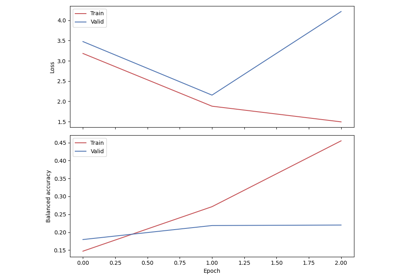
Sleep staging on the Sleep Physionet dataset using Eldele2021
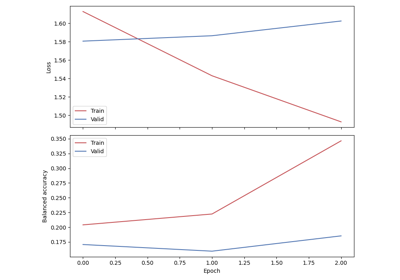
Sleep staging on the Sleep Physionet dataset using U-Sleep network